Tag: active research
Using LIDAR to assess the roles of climate and land-cover dynamics in biodiversity changes
Collaborators: Dr. Giorgos Mountrakis, Dr. Bill Porter, Dr. Colin Beier, Dr. Ben Zuckerberg, Dr. Lianjun Zhang, Dr. Bryan Blair Funders: NASA Biodiversity Program Motivation: Recent work has demonstrated a northern shift in bird habitats over the past 20 years in NY State. Our motivating question is to assess whether this […]
Read MoreA GIS-based urban growth model for Denver, Colorado
Collaborators: Dr. Giorgos Mountrakis, Jida Wang, Dr. Dimitrios Triantakonstantis Funders: NSF, NASA, SUNY ESF Graduate Assistantship Motivation: Urbanization is an important issue concerning scientists from different disciplines such as urban planners, biologists, engineers and is also extensively considered by policy-makers. A computational model quantifying locations and quantities of urban growth […]
Read MoreA multi-scale radial basis function neural network
Collaborators: Dr. Giorgos Mountrakis Funders: University of Maine covered patent application fees. Motivation: One of the constraints of radial basis function (RBF) neural networks revolves around the ability to combine local functions of variable spreads. Development of a multi-scale RBF would allow RBFs to exhibit their full potential in regression […]
Read MoreSatellite image classification by integrating multiple AI methods
Collaborators: Dr. Giorgos Mountrakis, Lori Luo, Dr. R. Watts Funders: Multiple sources. Motivation: Remote sensing as a field of study has reached its adulthood; computer-assisted classifiers have been in development for more than two decades. The complexity of remote sensing classification has led to a variety of methods, some of […]
Read More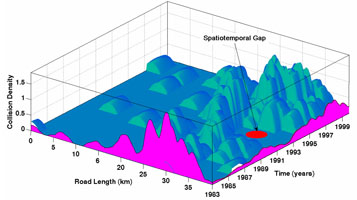
Spatiotemporal analysis on animal-vehicle collisions
Collaborators: Dr. Giorgos Mountrakis, Kari Gunson Funders: Humane Society, SUNY ESF Graduate Assistantship Motivation: Moose-vehicle collisions (MVCs) pose a serious safety and environmental concern in many regions of Europe and North America. For example, in the state of Vermont one-third of all reported MVCs resulted in motorist injury or fatality […]
Read More